What Is the Symbol Used to Represent the Sample Mean?
In statistics, you’ll encounter ‘x̄’ (pronounced ‘x-bar’) as the symbol representing the sample mean. This notation is pivotal when summarizing or analyzing sample data.
It’s calculated by summing all observations in your sample and dividing by the number of observations. Unlike ‘μ’, which denotes the population mean, ‘x̄’ focuses on sample subsets, providing insights into broader datasets.
Originating from early 20th-century statistical practices, ‘x̄’ underscores the evolution of data analysis, enabling precise decisions and inferential statistics applications. Grasping its significance paves the way to a deeper comprehension of data intricacies.
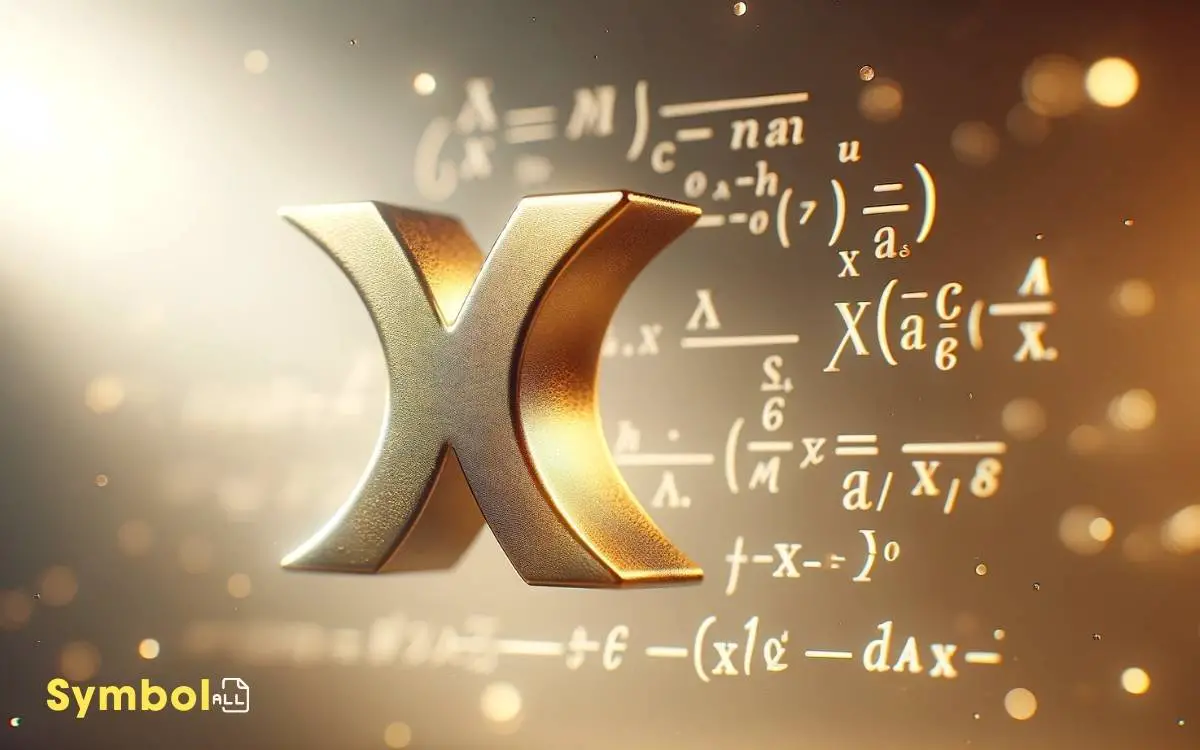
Key Takeaways
Understanding Sample Mean
The sample mean, a crucial statistic, represents the average value of a subset of a population. It’s calculated by adding all the values in the sample and then dividing by the number of values. This process yields a single value that summarizes the central tendency of the data.
You’re dealing with a tool that’s essential for making inferences about a larger population from a smaller group. It’s a cornerstone in the field of statistics, used extensively in both descriptive and inferential statistics.
Understanding the sample mean is fundamental because it lays the groundwork for more complex statistical analyses.
By grasping its concept, you’re better equipped to interpret data accurately, assess the reliability of your sample, and make informed decisions based on statistical evidence.
The Symbol Explained
You’ve encountered ‘X-bar‘ as the symbol for sample mean, but understanding its mathematical representation and origins clarifies its significance.
This symbol isn’t arbitrary; it’s rooted in statistical notation history, offering a precise method for denoting sample averages.
Grasping the rationale behind ‘X-bar’ equips you with a deeper comprehension of statistical analysis principles.
Understanding “X-bar”
In statistics, ‘X-bar’ symbolizes the sample mean, serving as a crucial indicator of central tendency in data sets.
Understanding ‘X-bar’ involves recognizing:
- Calculation: It’s derived by summing all observations in a sample and dividing by the sample’s size. This process highlights the average value, providing a snapshot of the data’s central point.
- Application: ‘X-bar’ is pivotal in inferential statistics, where it’s used to estimate the population mean from a sample. This estimation facilitates hypothesis testing and confidence interval construction.
- Interpretation: The value of ‘X-bar’ allows for comparisons between different data sets or within the same data set over time, offering insights into trends, consistencies, or discrepancies.
Grasping ‘X-bar’ is fundamental for anyone looking to analyze data with precision and technical acumen.
Mathematical Representation Origins
Often, people overlook the rich history and mathematical rigor behind the symbol ‘X-bar’, not realizing its origins are deeply rooted in statistical theory and notation. This symbol embodies the concept of averaging a set of values, a foundational aspect of descriptive statistics.
The ‘bar’ atop the ‘X’ denotes the arithmetic mean of a sample, distinguishing it from the population mean represented by the Greek letter ‘μ’ (mu).
Symbol | Represents | Origin |
---|---|---|
X-bar | Sample mean | 20th-century statistics |
μ | Population mean | Ancient Greek mathematics |
Σ | Summation | Late 19th-century calculus |
n | Sample size | Principally algebraic |
N | Population size | Principally algebraic |
Understanding ‘X-bar’ transcends mere familiarity; it requires an appreciation of its historical significance and mathematical precision.
Historical Origins
The symbol for sample mean, denoted as ‘x̄’, originated from the mathematical practices of statisticians in the early 20th century. This evolution reflects a period where statistical analysis began to solidify its role in research and data interpretation.
Several key developments contributed to its adoption:
- Standardization Need: As statistical methods gained prominence, a clear, universal symbol for sample mean was necessary for consistent communication among statisticians.
- Symbolic Efficiency: ‘x̄’ offers a concise representation, combining the variable of interest ‘x’ with a bar to indicate averaging, a practical solution for written and published works.
- Mathematical Rigor: The early 20th century saw a push towards formalizing statistical notations, with ‘x̄’ emerging as a product of this movement, encapsulating both the essence of averaging and its application to sample data.
This symbol’s inception was more than a mere convenience; it was a pivotal moment in the maturation of statistical science, ensuring clarity and uniformity across diverse fields.
Importance in Statistics
Understanding the symbol for sample mean is crucial as it serves as the foundation for statistical analysis.
You’ll find it’s a key for interpreting data accurately, enabling you to draw meaningful conclusions from datasets.
This knowledge allows you to evaluate the central tendency of data, a fundamental aspect in various fields of research and analysis.
Statistical Analysis Foundation
In statistics, grasping the foundation of statistical analysis is crucial for accurately interpreting data and making informed decisions.
This foundation rests on several key principles:
- Collection and Organization of Data: It’s essential to gather and structure data methodically to ensure its relevance and reliability for analysis.
- Descriptive Statistics: Understanding measures of central tendency, like the sample mean, and dispersion provides insights into data distribution and variability.
- Probability Theory: This underpins inferential statistics, allowing you to make predictions and generalizations about a population from sample data.
Mastering these elements enables you to apply statistical methods effectively, ensuring that your conclusions aren’t only data-driven but also statistically sound.
This knowledge is indispensable for navigating the complexities of data analysis and for the accurate interpretation of statistical results.
Data Interpretation Key
Mastering data interpretation is crucial, as it allows you to extract meaningful insights from statistical analysis, driving informed decision-making. When you delve into statistics, understanding the symbol for sample mean, often denoted by \( ar{x} \), is just the beginning.
You’re diving into a world where numbers narrate stories, trends, and patterns emerge from datasets, and every calculation holds the potential for impactful conclusions. It’s not merely about knowing the procedures but comprehending the outcomes’ significance.
Your ability to interpret these results accurately can significantly influence the strategies you recommend or the decisions you make.
In essence, data interpretation forms the bridge between theoretical statistics and practical application, enabling you to transform raw data into actionable intelligence. This skill is indispensable in a world inundated with information.
Calculating Sample Mean
To calculate the sample mean, sum all observed values and divide by the number of observations.
This process involves a few critical steps:
- Collect Data: Gather all the relevant observations or measurements that pertain to your study. Ensure accuracy and reliability in the data collection phase to prevent skewing the sample mean.
- Summation: Add up all the collected values. This total represents the cumulative value of your dataset, crucial for calculating the mean.
- Division: Divide the sum obtained in step 2 by the total number of observations in your sample. This final step yields the sample mean, symbolized as \(ar{x}\), providing a single value that serves as the central tendency of your dataset.
This methodological approach ensures precision in calculating the sample mean, offering a clear picture of your data’s central tendency.
Common Misconceptions
Many people mistakenly believe that the sample mean offers a complete picture of a dataset’s distribution, overlooking its potential limitations.
You must understand that while the sample mean provides a central tendency measure, it doesn’t account for the spread or variability within the data.
This misconception can lead you to underestimate the importance of other statistical measures like variance or standard deviation, which are crucial for a comprehensive data analysis.
Additionally, assuming that the sample mean can always represent the broader population can be misleading, especially if the sample isn’t randomly selected or is biased.
This misunderstanding highlights the necessity for a critical approach when interpreting statistical results, ensuring you don’t overlook the complexities inherent in data analysis.
Sample Mean Vs. Population Mean
Understanding the distinction between sample mean and population mean is crucial for accurate statistical analysis, as they serve different purposes in data interpretation.
Here are three key points to consider:
- Scope: The sample mean refers to the average of values in a subset of the total population, while the population mean covers every individual within the group.
- Symbolization: Typically, the sample mean is denoted by \( ar{x} \), whereas the population mean is symbolized by \( \mu \).
- Usage: You’ll use the sample mean when dealing with a manageable portion of data. It’s a practical approach to infer about the population mean, which is more theoretical and represents an ideal scenario where every data point can be considered.
Applications in Research
In research contexts, the application of sample means allows for the extrapolation of findings to larger populations with a degree of confidence.
This statistical tool is pivotal in hypothesis testing, where you compare the sample mean against a theoretical or known mean to ascertain differences or effects.
It’s also instrumental in constructing confidence intervals, offering a range within which the true population mean is likely to lie, based on your sample data.
Furthermore, sample means serve as the foundation for regression analyses, aiding in the prediction of dependent variables from one or more independent variables.
Interpretation Challenges
You often encounter interpretation challenges when dealing with the symbol for sample mean, particularly in misreading statistical notation. Variability in mean values adds a layer of complexity, requiring a nuanced understanding of the data.
Communicating these technical details effectively demands precision to avoid misconceptions.
Misreading Statistical Notation
Misreading statistical notation often leads to significant interpretation errors, especially when analyzing sample means.
In your quest for understanding, it’s critical to recognize common pitfalls:
- Confusing Symbols: The symbol \( ar{x} \) represents the sample mean. However, it’s easy to confuse this with \( \mu \), which denotes the population mean. This mix-up can drastically alter the interpretation of data.
- Overlooking Subscripts: Subscripts aren’t decorative; they specify the particular sample or variable in question. Ignoring them can lead to misapplying data insights.
- Misinterpreting the Context: Without a firm grasp of the context in which the sample mean is used, you might misapply statistical methods or draw incorrect conclusions about the dataset.
Understanding these nuances ensures a more accurate analysis of statistical data.
Variability in Mean Values
Understanding the variability in mean values presents a complex challenge, as it requires a precise analysis of how sample means can differ significantly from one another and from the population mean.
This variability, often quantified by measures such as standard deviation or variance, highlights the dispersion around the mean.
Factor | Impact on Variability |
---|---|
Sample Size | Larger sizes reduce variability |
Population Diversity | Greater diversity increases variability |
Measurement Precision | Higher precision decreases variability |
You’ll encounter situations where even with a robust methodology, the resulting sample means can show considerable spread due to these factors.
Grasping this concept is crucial; it underscores the importance of carefully selecting sample sizes and understanding population characteristics to accurately interpret mean values.
Communicating Technical Details
Although accurately interpreting the variability in mean values is foundational, conveying these technical details to a non-specialist audience poses significant challenges. You’re faced with the task of making complex statistical concepts accessible without diluting their significance.
Here are key points to consider:
- Language Use: You must choose terminology that’s both precise and comprehensible, avoiding jargon that can alienate or confuse.
- Visual Aids: Incorporating charts or graphs can elucidate data trends and statistical relationships, making abstract concepts more tangible.
- Contextualization: It’s critical to relate technical findings back to real-world implications, helping your audience grasp why these details matter.
Sample Mean in Software
In software, calculating the sample mean is a fundamental operation that often involves specific functions or algorithms to accurately aggregate data points.
To achieve this, you’ll typically leverage built-in statistical functions available in programming languages or software libraries.
For instance, in Python, the `numpy.mean()` function allows you to compute the sample mean with high precision by passing an array of data points as an argument. Similarly, in R, the `mean()` function serves this purpose.
These functions work by summing all data points in the sample and then dividing by the number of points, applying the formula for the arithmetic mean.
It’s crucial to ensure data integrity before calculation, as outliers or incorrect data can significantly skew the result.
Enhancing Data Analysis Skills
To enhance your data analysis skills, you’ll need to master various statistical methods and tools, ensuring accurate and insightful interpretations of data sets.
Here are critical steps to advance your proficiency:
- Learn Statistical Software: Familiarize yourself with software like R, Python, or SAS. Proficiency in these tools allows for efficient data manipulation, analysis, and visualization.
- Understand Statistical Techniques: Grasp the fundamentals of descriptive and inferential statistics, hypothesis testing, and regression analysis. Knowing when and how to apply these techniques is crucial.
- Practice on Real Datasets: Apply your knowledge to real-world datasets. This practice enhances your ability to discern patterns, make predictions, and validate hypotheses, thereby sharpening your analytical prowess.
Conclusion
In the vast landscape of statistics, the sample mean, symbolized by \( ar{x} \), stands as a beacon, guiding researchers through the fog of data. This symbol, rooted in historical mathematics, is crucial for distilling vast seas of information into digestible insights.
Calculating it’s akin to navigating the stars, using precise, analytical methods to chart a course through research inquiries. Yet, challenges in interpretation lurk like hidden reefs, demanding sharp skills to navigate.
Mastering \( ar{x} \) unlocks the power to illuminate the unknown, enhancing your data analysis voyage.