The Symbol Used for the Variance of the Sample Is? ‘s²!
In statistical analysis, you’ll encounter the need to quantify how data points deviate from the mean. To represent the variance of a sample, you use ‘s²‘.
This notation is fundamental in showcasing the extent of data spread around its average, allowing for an accurate evaluation of variability. It’s crucial for subsequent calculations like standard deviation, forming the backbone of inferential statistics and hypothesis testing.
Recognizing ‘s²’ as the symbol for sample variance facilitates your comparative analysis and enhances the precision of your findings. Embracing this understanding opens up further insights into data behavior and its implications.
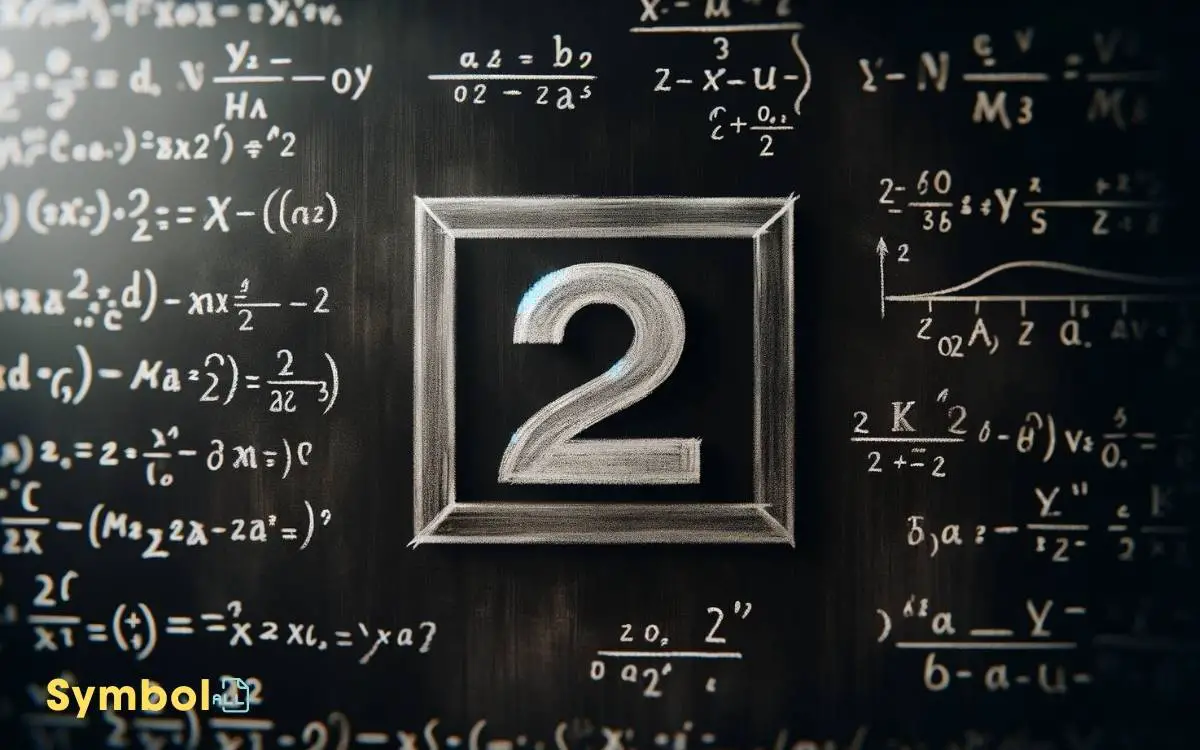
Key Takeaways
Understanding Variance
At its core, variance quantifies the dispersion of data points in a dataset, measuring how far each number in the set is from the mean. You’ll find it indispensable when you’re trying to grasp the spread of your data.
To calculate it, you first determine the mean of the dataset. Then, you subtract the mean from each data point, square these differences, and finally, average these squared differences.
This process isn’t just a mathematical exercise; it’s a fundamental step in understanding the variability within your data.
Importance of Sample Variance
Delving into the realm of sample variance sheds light on how data points in a sample deviate from the sample mean, offering crucial insights into the dataset’s spread and consistency. Understanding this measure is vital for you as it quantifies variability within your sample.
A low variance indicates that your data points tend to be close to the mean, suggesting a degree of uniformity in your dataset. Conversely, a high variance signifies a wide spread of data points, reflecting heterogeneity or potential outliers.
This knowledge is indispensable for statistical analysis, enabling you to make informed decisions based on the sample’s characteristics.
It aids in hypothesis testing, confidence interval construction, and in understanding the reliability of your data, serving as a cornerstone for further statistical inferences.
The Symbol Defined
In statistics, the symbol ‘s²’ represents the variance of a sample, offering a precise measure of how individual data points in your sample deviate from the sample mean. This symbol is pivotal in statistical analysis, enabling you to understand the distribution of data points effectively.
- Precision: ‘s²’ quantifies the degree of spread in your data, allowing for accurate interpretations.
- Comparative Analysis: Facilitates comparison between different data sets.
- Foundation: Essential for calculating standard deviation.
- Insightful: Offers insights into data variability.
- Analytical Tool: A critical component in hypothesis testing and inferential statistics.
Understanding ‘s²’ equips you with the ability to dissect and interpret your data’s behavior, making it a cornerstone of statistical analysis.
Historical Context
Understanding the variance symbol ‘s²’ offers valuable insights into data analysis; now, let’s explore its historical origins to appreciate its development and significance in statistics.
The concept of variance, integral to the field of statistics, emerged from the need to quantify the dispersion of data points around their mean.
Sir Ronald A. Fisher, a key figure in the development of modern statistical theory, introduced the term ‘variance’ in a 1918 paper, solidifying its foundational role.
Fisher’s work provided a rigorous method for assessing data variability, which is crucial for understanding the spread and reliability of data sets.
The notation ‘s²’ specifically denotes the sample variance, distinguishing it from the population variance symbolized by ‘σ²’. This distinction is vital for statistical analysis, guiding researchers in applying the correct formulae and interpretations.
Calculation of Variance
You’ll first need to grasp the variance formula, a critical step in quantifying the spread of data points around the mean. This involves squaring the differences between each data point and the mean, then averaging these squares.
Next, we’ll explore practical examples to illustrate how variance is applied in real-world scenarios, enhancing your understanding of its utility and calculation.
Variance Formula Explanation
To calculate the variance of a sample, subtract the mean from each data point, square the results, and then average those squared differences. This procedure quantifies the spread of your data, offering a clear measure of variability within your sample.
- Subtraction of the mean: Centers your data, facilitating comparison.
- Squaring differences: Neutralizes negative values, emphasizing larger deviations.
- Averaging: Distributes the squared deviations equally, providing a fair representation.
- Sample variance symbol: Denoted by \(s^2\), it distinguishes from population variance (\(\sigma^2\)).
- Precision: This method ensures a detailed insight into your data’s dispersion, aiding in more accurate statistical analysis.
Understanding this formula allows you to dissect your data’s behavior meticulously, laying a foundation for robust statistical inference.
Practical Variance Examples
Let’s dive into several practical examples to demonstrate how variance is calculated, further clarifying this statistical concept’s application.
Imagine you’ve collected data on daily sales in dollars for a week: $200, $150, $300, $250, and $100. Firstly, you’d calculate the mean (average) of these sales, which is $200.
Next, you subtract the mean from each data point, square the result, and then sum up these squared differences. The calculations would be: $(200-200)^2 + (150-200)^2 + (300-200)^2 + (250-200)^2 + (100-200)^2 = 0 + 2500 + 10000 + 2500 + 10000. Summing these gives $22500.
Now, since we’re dealing with a sample, you divide by one less than the total number of data points, which in this case is 4, yielding a variance of $5625. This meticulous approach underscores the variability within your dataset.
Variance Vs. Population Variance
Understanding the difference between sample variance and population variance is crucial for accurately analyzing statistical data.
- Scope: Sample variance measures variability within a sample, while population variance assesses it across an entire population.
- Formula Differences: Sample variance uses \(n-1\) in the denominator to correct for bias, unlike the population variance formula, which uses \(n\).
- Purpose: Sample variance helps estimate population variance when the whole population data isn’t available.
- Interpretation: High variance in a sample could suggest a wider data spread than in the population, depending on the sample’s representativeness.
- Usage: Researchers often rely on sample variance in inferential statistics to make predictions about the population.
These distinctions are essential for your understanding and application of statistical analyses in real-world scenarios.
Symbol Significance
Understanding the symbol for variance of a sample, typically denoted as \(s^2\), is crucial for your grasp of statistical analysis.
It serves as a mathematical representation that simplifies the process of quantifying variability within a sample.
Recognizing its importance allows you to accurately interpret and analyze data, highlighting the symbol’s pivotal role in statistics.
Mathematical Representation
In statistics, the symbol \(s^2\) represents the variance of a sample, encapsulating the average squared deviation from the mean of the sample. This measure provides insight into the dispersion or spread of data points within a sample, reflecting the variability around the sample mean. It is distinct from the population variance, which is typically denoted by \(\sigma^2\) and applies to an entire population rather than just a sample. Similarly, the symbol for population correlation coefficient, often represented by \(\rho\) (rho), describes the strength and direction of the linear relationship between two variables across a whole population.
This symbol is pivotal in conveying the dispersion within a dataset, offering a quantitative measure that’s crucial for inferential analysis.
- Precision: It quantifies variability with exactitude.
- Comparison: Facilitates comparing dispersion across datasets.
- Foundation: Underpins further statistical calculations, like standard deviation.
- Insight: Offers insights into data spread, beyond average values.
- Reliability: Enhances understanding of data reliability and consistency.
Statistical Analysis Importance
Grasping the significance of the symbol \(s^2\) propels your statistical analysis from basic to advanced, allowing for a deeper dive into the data’s inherent variability.
Understanding \(s^2\) equips you with the ability to quantify the dispersion within your sample, a fundamental step in ensuring the reliability of your conclusions.
This measure, variance, doesn’t just convey the spread; it lays the groundwork for computing standard deviation, a critical parameter for interpreting data spread in relation to the mean.
When you’re equipped with this knowledge, you’re better positioned to identify outliers, understand data distribution, and make predictions with higher accuracy.
This isn’t just about crunching numbers; it’s about unlocking the stories hidden within your data, enabling informed decision-making and fostering a robust analytical mindset.
Common Misinterpretations
Many people mistakenly interpret the symbol for the variance of a sample as representing the population variance, overlooking critical differences between these two statistical measures. This confusion can lead to significant misinterpretations when analyzing data.
To clarify:
- Sample variance uses a denominator of n-1, adjusting for bias in finite samples.
- Population variance employs the total number of data points (N) as its denominator.
Not distinguishing between these can lead to incorrect data analysis conclusions.
- Sample variance is typically denoted by \(s^2\), whereas population variance uses \(\sigma^2\).
- Understanding the difference is crucial for accurate statistical inference.
Variance in Statistical Analysis
Diving into the realm of statistical analysis, you’ll find that variance serves as a foundational measure, quantifying the spread of a dataset‘s numbers around their mean.
To calculate it, you subtract the mean from each data point, square the results, and then average these squares. This calculation offers a clear, mathematically rigorous method for assessing variability within a dataset.
It’s crucial because it lays the groundwork for further statistical analysis, including the calculation of standard deviation, which provides a more intuitive measure of spread by returning to the original units of data.
Understanding variance is pivotal; it enables you to grasp the diversity and distribution within your data, setting a solid base for complex statistical inferences, without stepping into the practical application realm yet.
Application in Research
In your research, understanding the symbol for variance of the sample is crucial for enhancing data accuracy.
It allows you to quantify the variability within your dataset, thereby identifying underlying trends more effectively.
This precision in analysis directly impacts the reliability and validity of your findings, making it an indispensable tool in empirical investigations.
Enhancing Data Accuracy
To enhance data accuracy in research, you must meticulously apply the symbol for variance of a sample as it directly impacts the reliability of the findings.
Precise calculation and application of this statistical measure ensure that your analysis reflects true variability within your data, thereby bolstering the credibility of your research outcomes.
- Ensure correct identification and application of the sample variance symbol (s²).
- Cross-validate calculations with software or statistical tools to minimize human error.
- Understand the underlying assumptions of variance to apply it appropriately in context.
- Regularly review statistical methodologies to stay updated on best practices.
- Integrate robust data cleaning procedures to eliminate outliers and errors before variance calculation.
Adhering to these strategies will significantly enhance the accuracy and reliability of your research data, fostering trust in your findings.
Identifying Trends
Identifying trends within research data requires a keen eye for patterns and anomalies that could signal underlying phenomena. You’ll often deal with vast datasets where the initial observation doesn’t readily reveal the trends at play.
To navigate this complexity, you’ll apply statistical tools to quantify variability and central tendencies, essentially using the variance symbol as a guide to measure dispersion. This process allows you to discern patterns that might otherwise remain obscured.
Employing regression analysis further aids in understanding the relationship between variables, providing a clearer picture of potential trends.
It’s critical to approach this analysis with a hypothesis-driven mindset, ensuring that you’re not merely identifying patterns but also evaluating their significance and potential impact on your research findings.
Challenges in Calculation
Calculating the variance of a sample presents several challenges, including the risk of computational errors and the need for precise data handling.
When you’re tackling this task, you’re likely to encounter a series of hurdles that can impact the accuracy and reliability of your results.
- Small Sample Sizes: Limited data can skew results, making variance appear larger or smaller than it truly is.
- Outliers: Extreme values can disproportionately influence the variance, leading to misleading conclusions.
- Data Entry Errors: Even minor mistakes in data entry can significantly alter variance calculations.
- Arithmetic Complexity: The formula for variance involves squared differences, increasing the risk of computational errors.
- Assumptions About Population: Incorrectly assuming a sample perfectly represents a population can lead to errors in estimating variance.
Understanding these challenges is crucial for accurate variance calculation and interpretation.
Software and Variance
You’ll find that variance calculation tools within statistical software offer robust features for handling complex data analysis techniques. These tools are designed to automate the process, ensuring precision and efficiency in your calculations.
Variance Calculation Tools
In today’s digital era, various software tools have emerged to simplify the process of calculating sample variance, offering precise and quick results. These tools leverage advanced algorithms and user-friendly interfaces to ensure accuracy and efficiency in statistical analysis.
Whether you’re a student, researcher, or data analyst, understanding the capabilities of these tools is crucial for your work.
- Excel: Offers built-in functions like VAR.S for sample variance calculation.
- R: A programming language with packages such as `stats` for statistical computing.
- Python: Utilizes libraries like NumPy to compute variance efficiently.
- MATLAB: Known for its powerful numerical computing environment.
- SPSS: A comprehensive tool favored for its advanced statistical analysis features.
Each tool has its unique strengths, making them indispensable for analyzing and interpreting data with precision.
Statistical Software Features
As you explore the landscape of statistical software, it’s imperative to understand how each platform’s unique features can streamline the process of computing sample variance. The intricacies of these features are central to performing accurate and efficient analysis.
Below is a comparative table that highlights key functionalities related to variance computation across different software:
Software | Variance Computation Feature |
---|---|
R | `var()` function |
Python (NumPy) | `numpy.var()` method |
Excel | VAR.S formula |
SPSS | Descriptive statistics menu |
MATLAB | `var()` function |
Analyzing this table, it’s clear each platform offers specific functions or methods to compute sample variance, underscoring the importance of selecting the right tool for your data analysis needs.
Data Analysis Techniques
Understanding the features of statistical software for variance computation lays the groundwork for mastering data analysis techniques that leverage these tools effectively.
You’ll appreciate the nuances of sophisticated data analysis when you:
- Utilize software to calculate sample variance quickly, ensuring precise results.
- Interpret the software’s output to understand the variability within your dataset.
- Apply these insights to make informed decisions based on the data’s spread.
- Explore advanced features for more complex statistical analysis, beyond basic variance.
- Continuously update your skills as software evolves, staying ahead in data analysis.
This approach not only enhances your analytical capabilities but also empowers you to handle various data sets with confidence, making the most out of the statistical software at your disposal.
Enhancing Data Interpretation
To enhance data interpretation, it’s crucial to grasp the variance of a sample as it quantifies the dispersion of data points. Understanding variance allows you to assess the reliability of the data, making it indispensable for accurate analysis.
It’s not just about calculating an average; it’s about understanding how much data points deviate from that average. This knowledge enables you to make informed decisions based on the spread of the data, rather than just the central tendency.
In practice, this means you’re better equipped to identify outliers, understand the distribution of your dataset, and predict future trends with greater accuracy.
Being analytical and precise in calculating and interpreting variance will significantly improve your ability to draw meaningful conclusions from your data.
Future of Statistical Symbols
Building on the importance of statistical measures like variance for data analysis, the evolution of statistical symbols represents a significant aspect of future developments in data science.
As you delve deeper into this field, you’ll notice that the symbols we use aren’t just arbitrary; they’re evolving to meet the needs of increasingly complex analyses.
- Introduction of symbols for new statistical measures as data complexity increases
- Standardization across software platforms to ensure consistency in data analysis
- Enhanced symbols for clearer representation of multi-dimensional data
- Adoption of interactive symbols in digital platforms for dynamic data exploration
- Development of universally accessible symbols to bridge the gap between different fields of research
These advancements are crucial for you to grasp the nuances of future data analysis and contribute effectively to the field.
Conclusion
You’ve embarked on a mathematical odyssey, uncovering the heart of data analysis: sample variance, symbolized by \(s^2\). This tiny titan of symbols unlocks the mysteries within datasets, serving as a beacon for researchers navigating the tumultuous seas of statistical analysis.
Its calculation, though intricate, is the linchpin for enhancing data interpretation, promising a future where statistical symbols continue to evolve, radically transforming our understanding of the world’s data. Embrace \(s^2\), for it’s your compass in the vast universe of numbers.