What Is the Symbol Used for the Population Correlation Coefficient?
The symbol you’re looking for, representing the population correlation coefficient, is ρ (rho). This Greek letter ranges from –1 to 1 and plays a pivotal role in illustrating the strength and direction of the relationship between variables.
Understanding ρ is not just about prediction; it’s essential in the realms of reliability and hypothesis validation within research contexts.
Through its employment in data analysis, ρ ensures accurate result interpretation, relying heavily on covariance and standard deviations in its calculation.
Its conception, deeply rooted in statistical history, has evolved to become a critical marker for linear relationship measurement. Exploring further unveils its broader implications and applications.
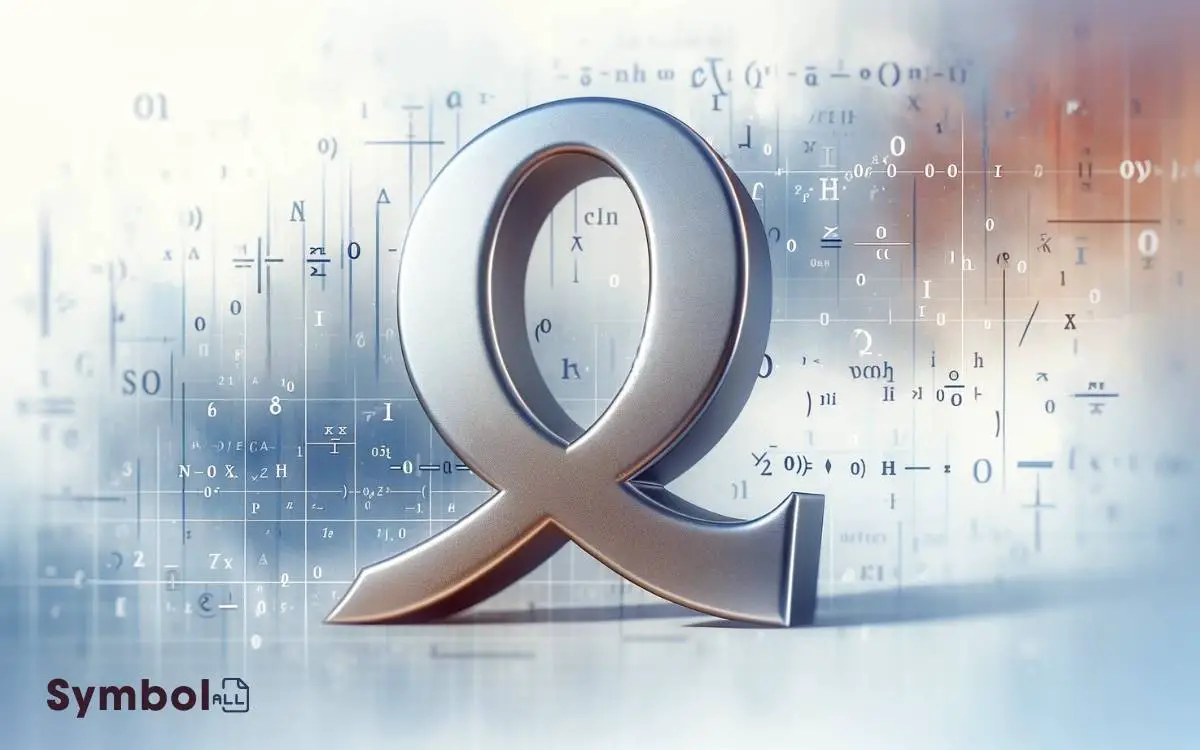
Key Takeaways
Defining Population Correlation
Understanding the concept of population correlation, you’ll grasp how two variables within a given population interrelate, providing insights into their statistical relationship. This measure quantifies the degree to which these variables move in tandem, either positively or negatively.
A positive correlation indicates that as one variable increases, the other tends to increase as well, whereas a negative correlation suggests that as one variable increases, the other tends to decrease.
It’s crucial to understand, however, that correlation doesn’t imply causation. This distinction means that while two variables may show a strong correlation, it doesn’t necessarily mean one causes the changes in the other.
Analyzing population correlation requires a meticulous approach, focusing on data collection, statistical methods, and the interpretation of results to ensure accuracy and relevance in your conclusions.
The Symbol Unveiled
The symbol representing the population correlation coefficient, denoted as \(\rho\) (rho), serves as a critical tool in quantifying the strength and direction of the relationship between two variables in a population.
You’ll find \(\rho\) at the heart of statistical analysis, where it ranges from -1 to 1.
A \(\rho\) value of 1 indicates a perfect positive correlation, meaning as one variable increases, so does the other.
Conversely, a \(\rho\) of -1 signifies a perfect negative correlation, where one variable’s increase corresponds to the other’s decrease. A zero value, on the other hand, implies no linear relationship.
Understanding \(\rho\) is essential, as it provides a foundation for making predictions, establishing reliability, and validating hypotheses in research across diverse fields.
Historical Origins
Delving into the historical origins of the population correlation coefficient, symbolized by \(\rho\), reveals its roots in the late 19th and early 20th centuries, marked by pivotal advancements in statistical theory and practice.
Year | Contributor | Contribution |
---|---|---|
1885 | Francis Galton | Introduced the concept of correlation |
1892 | Karl Pearson | Developed the correlation coefficient formula |
1904 | Charles Spearman | Introduced rank correlation |
1921 | Ronald A. Fisher | Refined correlation analysis |
These milestones laid the foundation for \(\rho\)’s modern application.
Spearheaded by Galton’s fascination with heredity, these intellectuals refined the mathematical treatment of relationships between variables, ultimately leading to the codification of \(\rho\) as a symbol for measuring the strength and direction of a linear relationship between two variables in a population.
Symbol Significance
You must grasp the importance of the population correlation coefficient symbol to fully appreciate its role in statistical analysis.
This symbol’s mathematical representation offers a concise way to convey the strength and direction of a relationship between two variables.
Understanding Symbol Importance
Understanding the significance of the population correlation coefficient symbol is crucial, as it quantitatively reflects the strength and direction of a linear relationship between two variables.
This symbol isn’t merely a placeholder; it’s a pivotal component in statistical analysis, embodying the essence of correlation in a concise form. In statistical theory, it often represents the strength and direction of the relationship between two variables, serving as a cornerstone for predictive modeling. Its use is just one example of how Greek symbols in statistics play a critical role in simplifying complex concepts and equations. By relying on these universally recognized notations, statisticians can communicate intricate ideas with precision and clarity across diverse fields of study.
Its presence in an equation or analysis signifies that the data under consideration has been investigated for linear correlation, offering insights into how one variable may predict or affect another.
Recognizing this symbol aids in comprehending the depth of analyses, ensuring you’re equipped to interpret results accurately.
It’s not just a symbol; it’s a beacon of relational insight, guiding you through the complexities of data analysis with precision.
Mathematical Representation Explained
Building on the foundation of symbol importance, let’s explore the mathematical representation of the population correlation coefficient to grasp its significance in statistical analysis.
Represented by the Greek letter ρ (rho), this coefficient quantifies the degree to which two population variables linearly relate to each other.
Its value ranges from -1 to 1, where 1 signifies a perfect positive linear relationship, -1 indicates a perfect negative linear relationship, and 0 suggests no linear relationship.
This calculation involves dividing the covariance of the two variables by the product of their standard deviations.
Understanding this formula is crucial as it provides a precise and quantifiable measure of the linear correlation between variables, enhancing your analytical capabilities in interpreting complex data sets.
Insights From Correlation Coefficient
Delving into the significance of the population correlation coefficient, symbolized by ρ, reveals its pivotal role in quantifying the linear relationship between two variables within a statistical framework. You’ll find ρ invaluable for discerning how closely two sets of data move in unison.
A perfect positive correlation, indicated by ρ = 1, means the variables increase or decrease together in perfect synchrony.
Conversely, ρ = -1 signifies a perfect negative correlation, where one variable increases as the other decreases. Notably, a ρ close to 0 suggests a lack of linear relationship.
Understanding ρ’s nuances enables you to predict outcomes, identify trends, and make informed decisions based on the strength and direction of relationships between variables.
This insight is crucial for researchers and analysts in various fields, enhancing the precision of their conclusions.
Calculating the Coefficient
To calculate the population correlation coefficient, you’ll first need to gather all pairwise data points from your dataset. This involves identifying each variable’s values across all observations.
You’ll then compute the mean for each variable, a crucial step for understanding their central tendencies. With these means, you’ll subtract each data point’s value from its variable’s mean, calculating deviations.
These deviations are pivotal for assessing the relationship’s strength and direction between variables.
Next, you’ll multiply corresponding deviations for each pair, summing these products. This sum is then divided by the product of each variable’s standard deviation, multiplied by the number of pairs minus one.
The result is the population correlation coefficient (ρ), quantifying the linear relationship between your variables, ranging from -1 to 1.
Common Misconceptions
Often, people mistakenly believe that a correlation coefficient close to 0 means there’s no relationship between the variables, overlooking the possibility of nonlinear connections.
This misconception stems from interpreting the correlation coefficient solely through the lens of linear relationships.
You must understand that the correlation coefficient measures the strength and direction of a linear relationship between two variables. However, complex relationships, such as quadratic or exponential, may not be captured effectively by this statistic.
Consequently, a near-zero value doesn’t conclusively indicate the absence of a relationship; it merely suggests that if a relationship exists, it mightn’t be linear.
To accurately assess relationships between variables, you should consider employing additional statistical tools designed to detect nonlinear patterns, thereby avoiding the pitfalls of oversimplified interpretations.
Symbol in Research
In research, the symbol for the population correlation coefficient, denoted as \(\rho\) (rho), plays a crucial role in quantitatively assessing the linear relationship between two variables.
This symbol is pivotal for several reasons:
- Standardization: \(\rho\) provides a universally recognized notation, facilitating communication among researchers worldwide.
- Precision: It allows for the precise quantification of the degree of correlation, serving as the foundation for further statistical analysis.
- Comparability: Using \(\rho\), findings can be directly compared across studies, enabling the synthesis of research outcomes.
Understanding \(\rho\) is essential for interpreting the strength and direction of relationships in your data. It’s not just a symbol but a gateway to unlocking insights within datasets, making it indispensable in your research toolkit.
Interpreting the Values
Having established the importance of the population correlation coefficient symbol \(\rho\) in research, let’s examine how its values can be interpreted to understand the strength and direction of the relationship between two variables.
The value of \(\rho\) ranges from -1 to 1. A \(\rho\) value of 1 indicates a perfect positive correlation, meaning as one variable increases, the other does likewise.
Conversely, a value of -1 signifies a perfect negative correlation, where an increase in one variable results in a decrease in the other. Values near 0 suggest a weak or no linear relationship.
It’s crucial to note that \(\rho\) doesn’t imply causation but merely quantifies the degree of linear association between variables. Understanding these nuances enables researchers to draw meaningful inferences from their data.
Software Representation
You’ll encounter various statistical software options when calculating the population correlation coefficient, each with its unique approach to computation and output interpretation.
Understanding how these tools represent and process the correlation coefficient is crucial for accurate analysis.
This knowledge enables you to critically assess software output, ensuring your interpretations align with the underlying statistical principles.
Common Statistical Software
Statistical software platforms, including R, Python, and SPSS, offer functionalities to calculate and interpret the population correlation coefficient, streamlining data analysis tasks.
These tools are indispensable in handling complex datasets and performing sophisticated statistical analyses.
Here’s how they stand out:
- R: Known for its extensive packages such as `cor()`, R facilitates complex statistical computations with ease, allowing for customizable scripts tailored to specific analysis needs.
- Python: With libraries like Pandas and SciPy, Python excels in data manipulation and statistical calculations, making it a go-to for data scientists looking for versatility.
- SPSS: Offers a user-friendly interface for non-programmers, with point-and-click options to compute correlations, thereby democratizing access to advanced statistical tools.
Each of these platforms offers unique advantages, catering to diverse user needs and proficiency levels in statistical analysis.
Calculating Correlation Coefficient
To calculate the population correlation coefficient, you’ll first need to select the appropriate function or library in your chosen statistical software. Most packages, like R, Python’s SciPy or Pandas, and MATLAB, offer built-in functions for this purpose.
In R, for instance, you’d typically use the `cor()` function, passing in your data vectors as arguments. Python users might opt for `pearsonr()` from SciPy or `corr()` when working with Pandas DataFrames. MATLAB enthusiasts can rely on the `corrcoef()` function.
It’s crucial to ensure your data meets the prerequisites for correlation analysis: linearity, homoscedasticity, and normality of the variables involved. Misinterpretation of these requirements can lead to inaccurate calculations, impacting your analysis’s validity.
Interpretation in Software Output
After calculating the population correlation coefficient using your preferred statistical software, interpreting the output becomes your next step. This crucial phase involves examining the numerical value provided, which ranges between -1 and 1.
Understanding this value is key to grasping the strength and direction of the relationship between your variables.
Here’s what to look for:
- Value Close to 1: Indicates a strong positive correlation; as one variable increases, so does the other.
- Value Close to -1: Signifies a strong negative correlation; one variable increases as the other decreases.
- Value Around 0: Suggests no linear correlation; the variables don’t move together in any specific, predictable way.
Interpreting these values accurately allows you to draw meaningful conclusions from your data analysis, guiding further research or decision-making processes effectively.
Comparative Analysis
When evaluating the effectiveness of the population correlation coefficient symbol, it’s crucial to compare its application across different statistical methodologies.
The symbol, often denoted as ρ (rho), serves as a foundation for understanding relationships between variables. However, its interpretation can vary slightly depending on the context.
Let’s dissect these nuances through a comparative table:
Methodology | Symbol | Interpretation |
---|---|---|
Pearson Correlation | ρ | Measures linear relationship |
Spearman’s Rank | ρ | Assesses monotonic relationship |
Partial Correlation | ρ | Evaluates the relationship, controlling for variables |
This table elucidates how the same symbol adapts to different statistical scenarios, highlighting its versatility.
Understanding these distinctions ensures you’re not only proficient in statistical theory but also in applying these concepts accurately in various research contexts.
Future Directions
Exploring future directions, it’s essential you understand how advancements in computational techniques might refine the application and interpretation of the population correlation coefficient symbol, ρ, across diverse datasets.
- Integration with Machine Learning Algorithms: Enhanced algorithms could enable more accurate predictions of ρ in complex, nonlinear relationships, providing insights previously unattainable.
- Big Data Analytics: The capacity to process and analyze larger datasets might reveal new patterns of correlation, altering our understanding of variables’ interdependencies.
- Improved Visualization Tools: Advanced tools could facilitate a more intuitive grasp of correlation matrices, making ρ’s implications clearer to researchers and decision-makers.
These developments promise a deeper, more nuanced comprehension of statistical relationships, ensuring that you’re at the forefront of leveraging ρ for sophisticated data analysis.
Conclusion
In your journey through the statistical cosmos, you’ve discovered that the symbol ρ (rho) serves as the beacon for population correlation, a measure whispering the strength of relationships between variables.
Grasping this concept and its calculation opens a portal to deeper insights, enabling you to navigate the data-driven landscape with precision.
As you wield this tool, remember, it’s not just about numbers; it’s about unveiling the hidden dialogues within the data, guiding your analytical endeavors into uncharted territories.