Greek Symbols Used in Statistics: Mu (µ), Alpha (α)!
In statistics, you frequently encounter Greek symbols such as Mu (µ) for population mean, which highlights central tendency, and Alpha (α) for the threshold of probability, essential in hypothesis testing decision-making.
Nu (ν) plays a critical role in statistical analysis, determining data variation constraints, while Beta (β) measures the likelihood of a Type II error, essential for precision in decision-making.
Lambda (λ) models arrival rates, important in operations research, and Theta (θ) denotes unknown parameters, pivotal in estimation techniques.
Each symbol carries significant analytical depth, guiding you through complex data interpretations. Venturing further offers a deeper understanding of their indispensable roles.
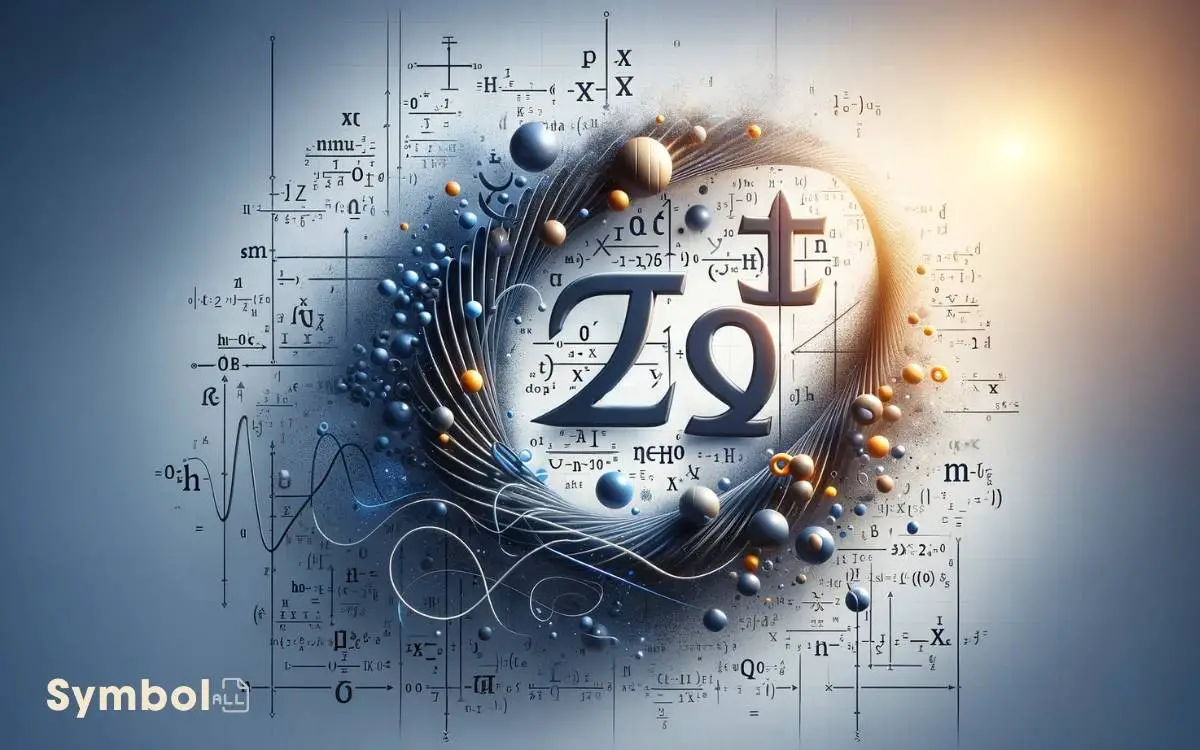
Key Takeaways
Understanding Sigma (σ)
Sigma (σ), a fundamental symbol in statistics, represents the standard deviation of a dataset, quantifying the variation or dispersion of the set’s values. Understanding σ is pivotal for discerning how spread out the numbers in your dataset are.
Fundamentally, it provides a clear measure of uncertainty or consistency within a set of data. You’ll use σ to identify outliers, understand data distribution, and make informed predictions.
When σ is low, you’re looking at a dataset with values tightly clustered around the mean. Conversely, a high σ indicates a wide range of values.
Mastering the interpretation of σ empowers you to critically analyze variability, enhancing your ability to draw meaningful conclusions from data. This analytical skill is indispensable in fields requiring precise data interpretation.
The Role of Pi (π)
After exploring the significance of sigma in quantifying data dispersion, we now turn our attention to pi (π), a symbol embodying the ratio of a circle’s circumference to its diameter. This constant, approximately equal to 3.14159, is fundamental not only in geometry but also in various branches of mathematics and science, appearing in formulas ranging from the area of a circle to Fourier transforms. Greek symbols frequently used in math, like sigma (σ) and pi (π), serve as universal shorthand for expressing complex concepts with precision and elegance. Their ubiquity highlights the enduring influence of ancient Greek contributions to modern mathematical thought.
In statistics, while pi may not have an essential role as it does in geometry, its value, approximately 3.14159, occasionally surfaces in formulas and equations that have underlying circular concepts or where normalization of distributions is involved.
You’ll find pi’s presence in statistical mechanics and in the calculation of areas or volumes where a circular dimension is integral. It’s important for you to grasp that pi’s utility extends beyond its geometric roots, subtly influencing statistical theory and practice.
Understanding its applications can enrich your comprehension of statistical methodologies, ensuring a more rounded approach to data analysis.
Alpha (α) in Hypothesis Testing
In the domain of hypothesis testing, alpha (α) serves as the pivotal threshold for deciding whether to reject the null hypothesis, marking an essential point in statistical analysis. You’re likely familiar with the concept, even if you don’t realize it.
Here’s why it’s vital:
- Threshold for Importance: Alpha determines the probability threshold under which the observed data is considered notably different from the null hypothesis.
- Risk of Error: It directly correlates with the risk of committing a Type I error, falsely rejecting the null hypothesis.
- Customizability: Researchers often set alpha at 0.05, but it’s adjustable based on the study’s tolerance for error.
Understanding alpha’s role helps you grasp the delicate balance between sensitivity and specificity in hypothesis testing. It’s not just a number; it’s a critical part of your decision-making process in statistical analysis.
Beta (β) and Type II Errors
As you shift from grasping the role of Alpha (α) in hypothesis testing, it’s essential to understand the concept of Beta (β) and how it’s interconnected with Type II errors.
Beta signifies the likelihood of committing a Type II error, which happens when you don’t reject a false null hypothesis.
This comprehension is pivotal in assessing the strength of statistical tests and their vulnerability to making inaccurate inferences.
Defining Beta (β)
Why is understanding Beta (β), or the probability of committing a Type II error, critical in statistical hypothesis testing?
- Precision in Decision-Making: Grasping the concept of Beta (β) equips you with the ability to precisely estimate the risk of incorrectly accepting the null hypothesis when, in fact, the alternative hypothesis is true.
- Resource Optimization: By understanding β, you’re better positioned to design studies or experiments that are both resource-efficient and have adequate power to detect true effects, avoiding unnecessary expenses and effort.
- Enhanced Interpretation: A solid grasp of β aids in the nuanced interpretation of statistical results, allowing for a more thorough understanding of the data and its implications within the context of the research question.
Understanding β isn’t just about avoiding a statistical faux pas; it’s about enhancing the credibility and impact of your research findings.
Understanding Type II Errors
Building on the understanding of Beta (β) enhances your grasp of Type II errors, where failing to reject a false null hypothesis has significant implications for research outcomes.
Essentially, a Type II error occurs when you’ve missed detecting a true effect; your study concludes there’s no significant finding when, in reality, there is.
This error is directly tied to the power of a test, which is 1-β. The higher the power, the lower the chance of committing a Type II error.
Carefully designing your study, including selecting an appropriate sample size and significance level, minimizes the risk of such errors. It’s pivotal to balance the risk of Type I and Type II errors, as this strategy safeguards the integrity and reliability of your research findings.
The Significance of Mu (μ)
In the domain of statistics, the symbol μ (mu) represents the mean or average of a population, serving as a cornerstone for various analytical methodologies.
Understanding μ is important for several reasons:
- Central Tendency: It gives you a quick glimpse into the center of your data set, helping to understand the general performance or behavior of the group being studied.
- Comparative Analysis: By knowing the average, you can compare different populations more accurately. This comparison is foundational in hypothesis testing, where you’re often looking to see if there’s a significant difference between groups.
- Predictive Analytics: μ plays a significant role in predictive models. It helps in setting the ground for forecasting future trends based on historical data, making it essential in decision-making processes.
Nu (ν) in Degrees of Freedom
You’ll find that understanding degrees of freedom is essential in statistical analysis, where nu (ν) plays a significant role. Nu’s function helps you grasp the constraints within which your data can vary, a cornerstone for accurate model interpretation.
Exploring practical applications provides insights into how these theoretical concepts impact real-world analyses, ensuring you’re well-equipped to apply statistical methods effectively.
Defining Degrees of Freedom
Understanding degrees of freedom, denoted as Nu (ν), is crucial when you’re analyzing statistical models to ensure their accuracy and reliability.
Degrees of freedom fundamentally represent the number of independent values or quantities that can vary in an analysis without breaking any constraints.
Here’s a breakdown:
- Independence: Each degree of freedom signifies an independent piece of information that can influence the outcome of your statistical calculations.
- Constraints: Degrees of freedom are influenced by constraints within the data. Subtracting the number of constraints from the total number of observations gives you the degrees of freedom.
- Variability: They allow for an understanding of how much variation can occur in your data, impacting the precision of your statistical estimates.
Grasping this concept is crucial for crafting and interpreting studies with a high degree of scientific rigor.
Nus Role Explained
Delving deeper, Nu (ν) plays a pivotal role in determining the degrees of freedom, directly impacting the reliability of statistical outcomes.
Essentially, the degrees of freedom concept is fundamental in statistics, providing insights into the amount of independent information within a dataset.
Nu’s involvement is critical, as it represents the number of independent pieces of information that go into the estimation of a parameter. This not only affects how you interpret the data but also influences the precision of statistical models.
Understanding Nu’s role allows you to discern how many observations are effectively contributing to the estimation process, affecting the robustness and generalizability of your findings.
It’s a delicate balance, where Nu guides the statistical power and accuracy of your analysis, ensuring that interpretations are both meaningful and reliable.
Practical Applications Insights
Exploring Nu (ν) in the context of degrees of freedom reveals its critical role in boosting the precision of statistical analyses. Understanding how ν operates can greatly enhance your grasp of statistical methods and their outcomes.
Here’s why it’s important:
- Model Accuracy: ν determines the flexibility of a statistical model, allowing it to fit the data more accurately without overfitting.
- Hypothesis Testing: In t-tests and chi-square tests, ν guides the selection of the correct distribution, which is essential for determining the p-value accurately.
- Confidence Intervals: The calculation of confidence intervals, especially in complex models, relies on ν to adjust the intervals’ width, ensuring they’re neither too conservative nor too optimistic.
Lambda (λ) for Arrival Rates
In statistics, lambda (λ) serves as the key parameter for quantifying arrival rates in various contexts. It’s crucial for you to grasp how λ operates within the framework of Poisson and exponential distributions, guiding predictions about how often events occur within a fixed interval.
Context | Role of λ |
---|---|
Queuing Theory | Models the rate of customer arrivals |
Traffic Flow | Measures car arrivals at a checkpoint |
Network Theory | Quantifies data packet arrivals |
Inventory Management | Estimates arrival of demand |
Reliability Engineering | Predicts failure rates |
Understanding λ allows you to analyze and predict patterns effectively, making it invaluable in operations research, engineering, and other fields that rely on forecasting event occurrences.
Its precision in estimating arrival rates guarantees strategies and systems are designed to accommodate real-world demands.
Epsilon (ε) and Margins of Error
You’ll find that epsilon (ε) plays a pivotal role in the domain of statistics, particularly in defining margins of error. Understanding how to calculate these margins accurately is essential for interpreting statistical results with confidence.
This discussion will guide you through the process, emphasizing the importance of precision in statistical analysis.
Epsilons Role
Epsilon (ε) plays a critical role in determining the margins of error, directly impacting the precision of statistical analyses. It’s a measure you can’t overlook if you’re aiming for accuracy in your findings.
Understanding ε’s influence involves recognizing a few key aspects:
- Epsilon’s Definition: It represents the maximum permissible error in the result of a calculation, setting the bounds for variability.
- Impact on Research: Smaller ε values suggest tighter confidence intervals, indicating more precise estimates.
- Statistical Significance: ε helps in evaluating the statistical significance of results, guiding researchers in making informed decisions.
Calculating Error Margins
To accurately assess error margins, you must investigate into the calculation of epsilon (ε), a critical step in ensuring the precision of your statistical analysis.
This process involves determining the range within which the true value of a parameter lies, with a certain level of confidence.
To calculate the margin of error, you’ll use the standard error of the statistic and a critical value based on the confidence level you desire.
This critical value is often derived from the Z-distribution or the t-distribution, depending on the sample size and whether the population standard deviation is known.
Theta (θ) for Unknown Parameters
In statistical analysis, theta (θ) commonly denotes an unknown parameter whose value is to be estimated. You’ll find θ pivotal in various statistical methods.
It represents a value that, while not directly observable, plays a key role in your analysis and conclusions. Understanding its role is important for accurate statistical modeling.
Here are three key points to keep in mind:
- Theta’s Versatility: Whether you’re dealing with means, proportions, or any other parameters, θ serves as a placeholder until actual values can be determined through data analysis.
- Estimation Techniques: Techniques like Maximum Likelihood Estimation (MLE) rely on θ to find the parameter value that makes the observed data most probable.
- Theta in Hypothesis Testing: It’s central in formulating null and alternative hypotheses, where you’ll test assumptions about θ’s true value.
Grasping the concept of θ enriches your statistical toolkit, enabling more precise and systematic research endeavors.
Rho (ρ) in Correlation Coefficients
Understanding the role of theta (θ) in statistical analysis prepares you to grasp the significance of rho (ρ) when evaluating correlation coefficients between variables. Rho represents the strength and direction of a linear relationship between two continuous variables.
It’s a measure that ranges from -1 to 1, where values closer to -1 or 1 indicate a strong correlation, and values near 0 suggest a weak or no linear relationship.
Symbol | Description | Relevance in Statistics |
---|---|---|
θ | Unknown Parameter | Basis for estimation and hypothesis testing |
ρ | Correlation Coefficient | Indicates the strength and direction of a linear relationship |
σ | Standard Deviation | Measures the dispersion of a dataset |
Understanding ρ’s role helps you analyze and interpret the dynamics between variables methodically and precisely, enhancing your statistical insights.
Phi (φ) and the Golden Ratio
Exploring the symbol Phi (φ), you’ll uncover its connection to the intriguing mathematical concept known as the Golden Ratio, a fundamental proportion deeply embedded in various aspects of nature and design.
This ratio, approximately equal to 1.618, isn’t only aesthetically pleasing but also appears in the arrangement of leaves, the patterns of galaxies, and the proportions of the human body.
Here’s how Phi (φ) relates to statistical analyses and understanding:
- Proportionality Analysis: It helps in understanding the natural proportions in data sets, especially in the field of design and architecture.
- Pattern Recognition: Phi can be used to identify patterns that follow the Golden Ratio, providing insights into natural and artificial systems.
- Optimization Problems: In optimization, the Golden Ratio can guide towards the most efficient and aesthetically pleasing solutions.
Omega (Ω) in Total Probability
Explore the concept of Omega (Ω) to reveal its essential role in calculating total probability, guaranteeing a thorough understanding of statistical outcomes. Omega represents the sample space, or the set of all possible outcomes in a statistical experiment.
Your grasp of Ω ensures you’re accounting for every possible event, a fundamental step in probability calculations.
Event | Probability | In Ω? |
---|---|---|
A | 0.2 | Yes |
B | 0.3 | Yes |
C | 0.5 | Yes |
D | 0 | No |
This table illustrates how events within Ω contribute to the total probability. Notice that Event D, with a probability of 0, isn’t considered part of the sample space Ω. Understanding this distinction sharpens your statistical analyses, making them accurate and all-encompassing.
Conclusion
In your statistical odyssey, you’ve encountered the pantheon of Greek symbols, each holding a unique power in deciphering the universe’s numerical riddles.
From Sigma’s embrace of variability to Theta’s veil over the unknown, these characters are more than mere notation they’re the keys to revealing profound truths, akin to Prometheus gifting fire to humanity.
As you wield these symbols, remember, your analyses don’t merely crunch numbers; they breathe life into data, exposing the cosmos’s hidden patterns.